
Increasing trust in ML through governance
Prof. Nicolas Papernot
University of Toronto
The attack surface of machine learning is large: training data can be poisoned, predictions manipulated using adversarial examples, models exploited to reveal sensitive information contained in training data, etc. This is in large parts due to the absence of security and privacy considerations in the design of ML algorithms. Designing secure ML requires that we have a solid understanding as to what we expect legitimate model behavior to look like. We illustrate these directions with recent work on adversarial examples, model stealing, privacy-preserving ML, machine unlearning, and proof of learning.
Speaker
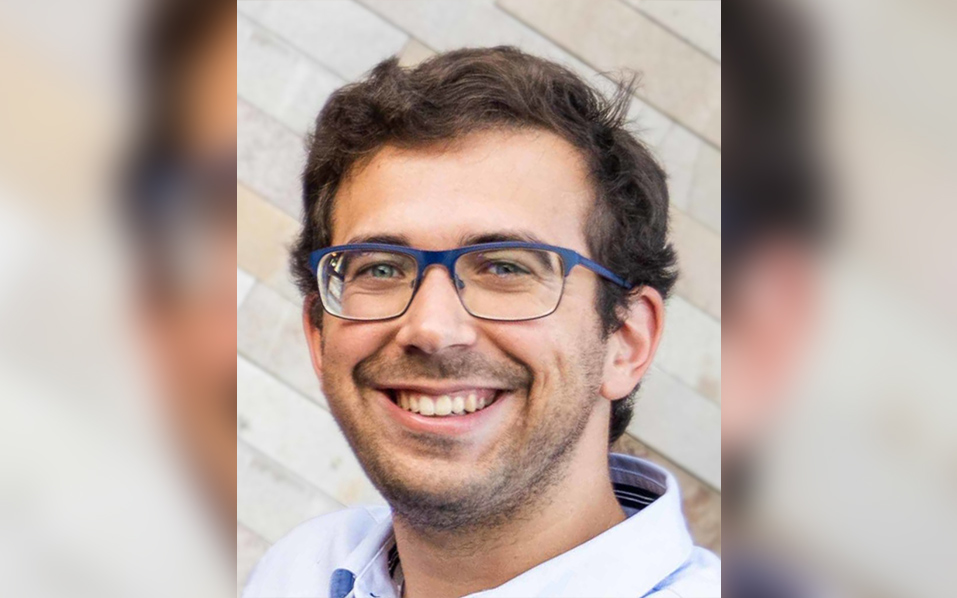
Prof. Nicolas Papernot
is an Assistant Professor in the Department of Electrical and Computer Engineering and the Department of Computer Science at the University of Toronto. He is also a faculty member at the Vector Institute where he holds a Canada CIFAR AI Chair, and a faculty affiliate at the Schwartz Reisman Institute. His research interests span the security and privacy of machine learning. Nicolas is a Connaught Researcher and was previously a Google PhD Fellow. His work on differentially private machine learning received a best paper award at ICLR 2017. He is an associate chair of IEEE S&P (Oakland) and an area chair of NeurIPS. He earned his Ph.D. at the Pennsylvania State University, working with Prof. Patrick McDaniel. Upon graduating, he spent a year as a research scientist at Google Brain.
is an Assistant Professor in the Department of Electrical and Computer Engineering and the Department of Computer Science at the University of Toronto. He is also a faculty member at the Vector Institute where he holds a Canada CIFAR AI Chair, and a faculty affiliate at the Schwartz Reisman Institute. His research interests span the security and privacy of machine learning. Nicolas is a Connaught Researcher and was previously a Google PhD Fellow. His work on differentially private machine learning received a best paper award at ICLR 2017. He is an associate chair of IEEE S&P (Oakland) and an area chair of NeurIPS. He earned his Ph.D. at the Pennsylvania State University, working with Prof. Patrick McDaniel. Upon graduating, he spent a year as a research scientist at Google Brain.